February 2021
Executive Summary
We examine the rise of technology from the perspective of its human creators. We measure companies’ investments in technical human capital to identify firms that are truly embracing the digital age. These intangible investments have generated strong stock returns across a wide variety of industries. We conclude with a discussion of the disruptive impact of technology on labor markets.
The Knowledge Economy
Digital Transformation
The Covid-19 pandemic is accelerating the digitization of the economy. In the past year, the adoption of e-commerce, cloud computing, remote work, telemedicine, and online education have increased from a trickle to a torrent.
Exhibit 1
Gradually, Then Suddenly
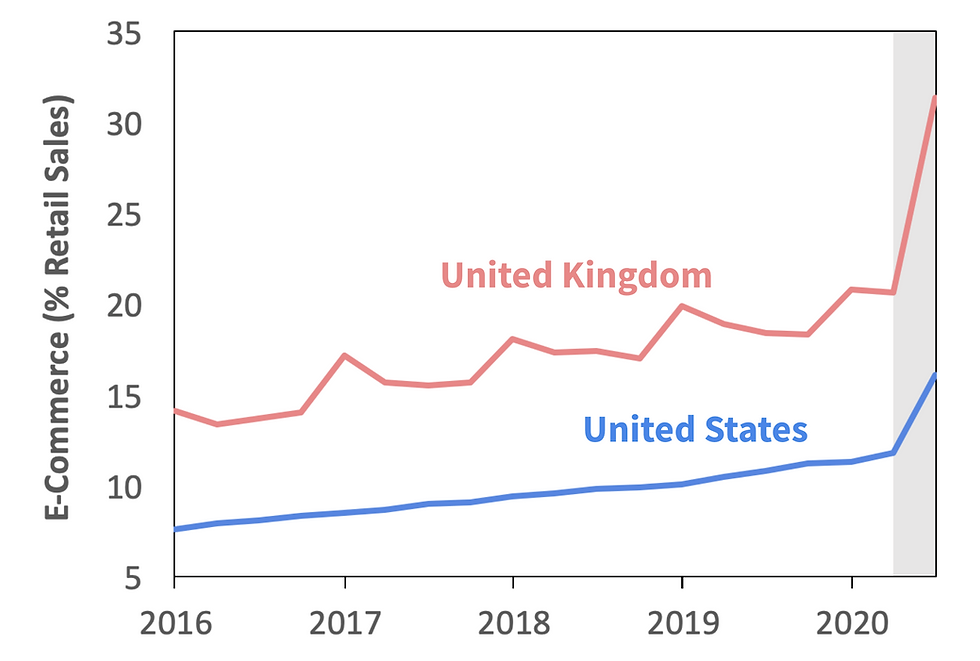
Source: US Census Bureau, UK Office for National Statistics, Sparkline
Companies that were already positioned for the digital age have predictably thrived. More importantly, the pandemic is forcing the mass market of established firms to “cross the chasm” and embrace the digital economy.
Exhibit 2
Crossing the Chasm
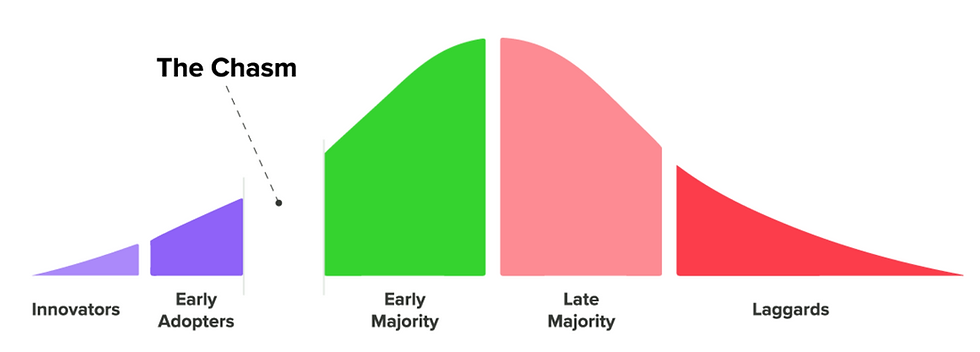
Source: Geoffrey Moore, New Breed
The Half-Life of Knowledge
While the pandemic may be a one-off event (or so we hope 🤞), it turns out that the adoption of new ideas has been accelerating for over a century. The diffusion of new inventions tends to follow an “S-curve” with a few early adopters taking the initial risk, followed by the mass market and eventually a handful of laggards.
Exhibit 3
S-Curve Compression

Source: Harvard Business Review, New York Times
Over the past century, S-curves have been compressing. The telephone took 40 years to achieve 40% adoption, the cell phone achieved this market penetration in around 20 years, and the smartphone did so in just 10 years.
In 1982, R. Buckminster Fuller coined the concept of the “knowledge doubling curve.” He estimated that up until 1900, human knowledge doubled every century. However, by 1945 knowledge doubled every 25 years and by 1982 it doubled every year. Not only is knowledge increasing exponentially, but its growth rate is accelerating.
The compounding of knowledge has enabled the human population to grow and flourish. However, its acceleration also implies that ideas are more quickly becoming obsolete. The half-life of knowledge is rapidly diminishing.
The knowledge we acquired in college, graduate school, and our last job may already be obsolete or even debunked. This is especially salient in fast-moving fields like technology. As futurist Alvin Toffler wrote, “the illiterate of the 21st century will not be those who cannot read and write, but those who cannot learn, unlearn, and relearn.”
The Knowledge Worker
“The most valuable asset of a 21st-century institution, whether business or non-business, will be its knowledge workers and their productivity.”
- Peter F. Drucker, Management Challenges for the 21st Century (1999)
The rapid growth and turnover of human knowledge has fueled the ascent of the knowledge worker. These people wield a combination of intellect and creativity to solve the complex, unstructured problems of the information age.
Knowledge workers’ contributions are highly scalable due to the scalability of information itself. It costs almost nothing to create additional copies of digital media or software. This gives extreme leverage to those at the top of their fields. Laszlo Bock, Google’s former HR head, wrote that, like stock returns, “individual performance also follows a power law distribution.” A top Google engineer can be 100 times as productive as the median one, a distribution unlikely to be found among assembly line workers, for example.
This dispersion implies that the ability for firms to identify, attract, retain, and nurture top talent is the key competitive advantage in the modern age. As Drucker (1994) wrote, “the basic economic resource - the means of production - is no longer capital, nor natural resources, nor labour. It is and will be knowledge.” And knowledge is embodied by people.
Human Capital Investment
The People-Heavy Economy
“Our people are our greatest asset.”
- Every CEO
In order to succeed in the information age, companies have ramped up their investment in human capital. This requires investing not only in hiring new talent but also in continually reskilling and upskilling existing employees as the world evolves.
In Investing in the Intangible Economy (Oct 2020), we discussed the effort by Corrado, Hulten and Sichel (2009) to incorporate intangible investment into national accounting. Human capital falls under “firm-specific resources,” defined as “employer-provided worker training and … management time devoted to enhancing the productivity of the firm.” As of 2003, this comprised 35% of intangible investment.
Exhibit 4
Intangible Investment
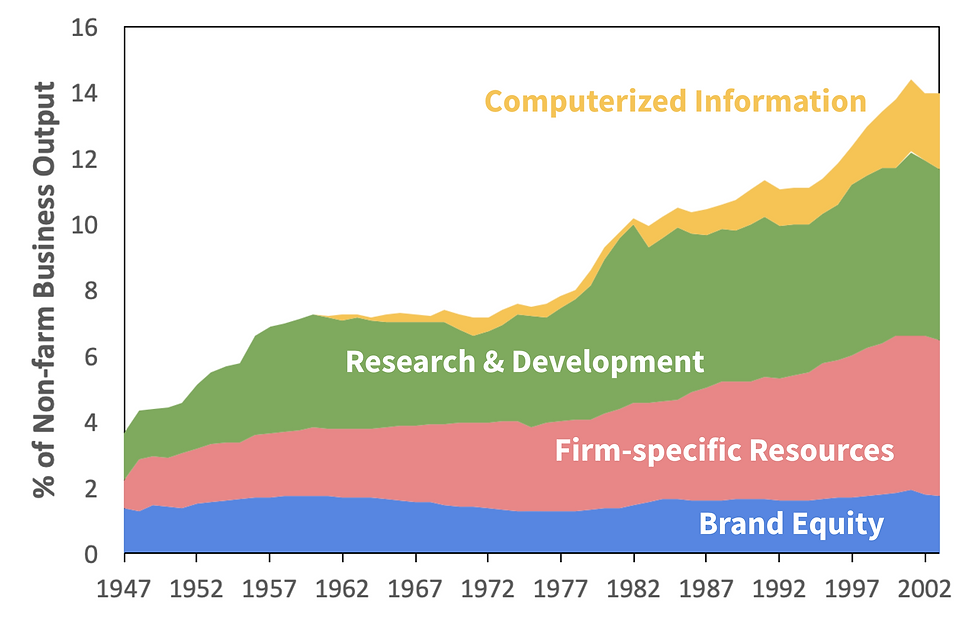
Source: Corrado, Hulten and Sichel (2009), Sparkline
Using a broader definition, Regier and Rouen (2020) take advantage of the fact that IFRS accounting standards (i.e., outside the U.S.) mandate the disclosure of personnel expenditures, which include hiring and termination costs, salaries and bonuses, benefits and perquisites, and training and development costs.
Exhibit 5
Our People Are Our Greatest Expenditure

Source: Regier and Rouen (2020), Sparkline
In the past 25 years, personnel expenditures have increased from 30% to 50% of sales. Meanwhile, capital expenditures have remained flat. Not only is the economy becoming asset-light, but it is becoming people-heavy!
Intangible Investing
In Investing in the Intangible Economy (Oct 2020), we introduced a style of investing we call “intangible investing.” The insight is that markets tend to undervalue companies making significant investments in intangible assets. One reason is that accounting standards have failed to adapt to the rising role of intangibles in the modern economy. More generally, intangible assets are challenging to value, which leads many investors to simply ignore them (at their peril).
As a result, buying intangible-rich companies has tended to produce market-beating returns. We documented this effect for companies that invest in R&D, advertising, patents, and brand equity. For our theory of “intangible investing” to be consistent, we would expect to find that firms investing heavily in human capital are similarly undervalued. Indeed, Regier and Rouen (2020) find that such companies have produced annual excess returns of 6.3 to 9.3%. Check ✅!
Exhibit 6
A Theory of Everything?

Source: Sparkline
Measuring Human Capital
Unfortunately, this study cannot be replicated in the U.S. due to our more limited accounting disclosures. Accounting reform has lagged the rise of the intangible economy.
But pressure from ESG proponents and many others is finally starting to bear fruit. In Aug 2020, after 30 years of essentially no changes, the SEC finally announced an attempt to modernize reporting with an “increased focus on human capital disclosures, which for various industries and companies can be an important driver of long-term value.” However, it still remains to be seen how exactly these vague materiality standards will be implemented and if this will lead to the consistent and broad disclosure of key human capital metrics in the future.
Thus, at least for now, we need to go beyond financial statements to truly understand companies’ human capital investments. Fortunately, while technology is accelerating the pace of labor market disruption, it is also providing researchers with powerful new ways to understand these changes.
We will consider two datasets. First, online job postings provide insight into companies’ labor demand. Job postings typically include a job title and location as well as required and preferred skills, experience, and education.
Exhibit 7
Job Posting Example
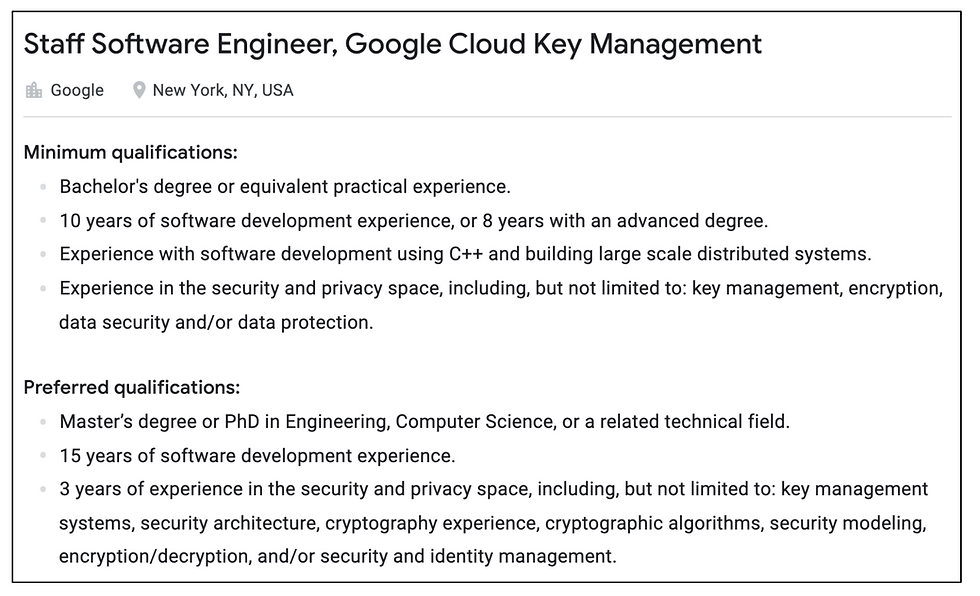
Source: Google, Sparkline
Second, digitized resumes contain detail on individual employees' experiences and skills. These data exist publicly in resume banks and social networks such as LinkedIn. They contain a wealth of information, such as employment history, education, technical skills, certifications, patents and other accomplishments.
Exhibit 8
LinkedIn Profile Example
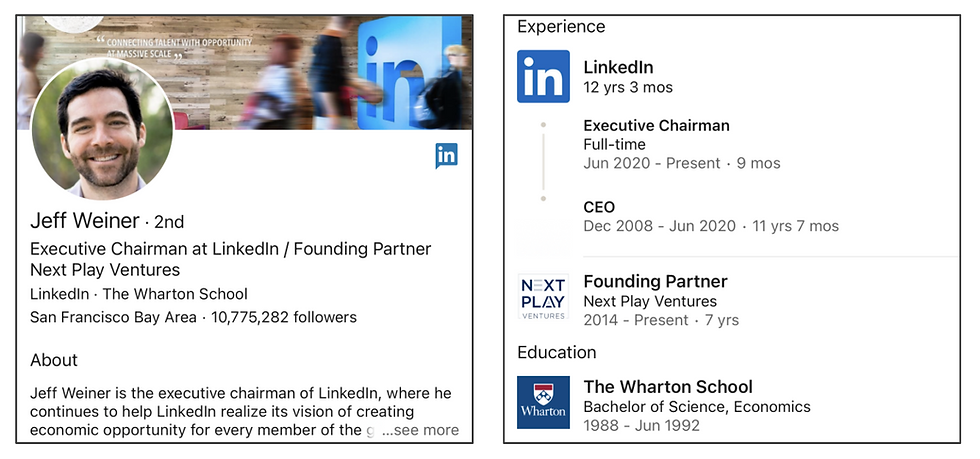
Source: LinkedIn, Sparkline
These sources complement each other, providing insight both into companies’ hiring intent and actual workforce composition. While they consist primarily of unstructured data (i.e., text), they can be parsed using simple natural language processing (NLP) tools.
Digital Transformation
People, Not Technology
“The existing assumption is that to digitally transform a company, you need to adopt the latest technologies. While this is partly correct, it doesn’t tell the whole story… Digital Transformation is just as much about people and organizational change as it is about the specific technologies being used.”
- Garth Andrus, Digital Transformation (2019)
It is widely recognized that established companies need to reposition themselves for the digital age. Even before the pandemic, 87% of respondents to a MIT survey recognized that their industry was likely to be disrupted by digital technologies. And Covid-19 has only intensified the urgency of digital transformation.
Over the past year, we have seen several legacy companies make great inroads in reorienting their businesses. For example, Best Buy and Target grew their e-commerce sales by high triple digits through the pandemic.
Exhibit 9
Old Dogs, New Tricks

Source: SEC
Unfortunately, these success stories are the exception rather than the rule. McKinsey (2018) estimates that fewer than 30% of digital transformations succeed. It is not as simple as plopping down $100 million for an enterprise software system. Success requires not just technology but leadership, employee training, and organizational restructuring. These complementary investments are essential to beating the odds and pulling off a successful digital transformation.
Follow the Talent
As investors, it is critical to assess how committed firms are to embracing modern paradigms. Companies that are not adapting to the digital age risk being disrupted. The challenge is that CEOs are incentivized to talk a big game in filings, calls, and press releases. Investment in technical talent provides a “ground truth” way to see if their money is where their mouth is. 🤑
There are three ways to identify technical talent. First, we can use job titles, such as “database administrator” or “devops engineer.” Second, we can look for roles that require technical skills, such as SQL, Linux, or TensorFlow. Third, we can look for jobs requiring computer science degrees. In all cases, the data point to an unambiguous surge in demand for technology workers.
More importantly, the surging demand for technical talent is not confined to the Information Technology (IT) industry. In fact, Markow, Coutinho, and Bundy (2019) find that 90% of tech job openings are outside the IT industry. Moreover, IT job demand has actually been growing more quickly outside of the IT sector than within it (+65% vs. +40%)
Exhibit 10
Not Just For Nerds 🤓

Source: Markow, Coutinho, and Bundy (2019), Sparkline
The technological revolution can no longer be confined to a single niche of the economy. Software is eating the world. All companies are now technology companies.
However, companies are at varying phases in this journey. Building on our earlier example, the next exhibit shows the share of retailers’ workforces in IT roles based on LinkedIn. As expected, purely online retailers such as Wayfair, eBay and Chewy currently sit at the top of this list. However, many traditional retailers are starting to catch up. 🚀
Exhibit 11
IT Employers in Retail

Source: S&P, LinkedIn, Sparkline
Educational Attainment 🎓
We have so far been purposefully generous in our definition of technology workers. Both Bill Gates (creator of Microsoft) and Bill Lumbergh (creator of the TPS report 📋) would be classified as “IT workers.” Therefore, we would like to create a more narrow definition for cutting-edge technical talent.
For better or worse, the bulk of human capital investment is made in formal education. All else equal, a PhD implies more expertise than a master’s degree, which signals more skill than a bachelor’s. Thus, educational attainment provides a convenient measure of skill level. Of course, this is only a very rough proxy: Bill Gates dropped out of college and is doing just fine!
Let’s measure the percentage of companies' IT workforces with PhDs. We’ll narrow our focus to include only PhDs in relevant fields of study. The most prolific employers of IT PhDs are in the IT and communications sectors (red).
Exhibit 12
PhD Employers
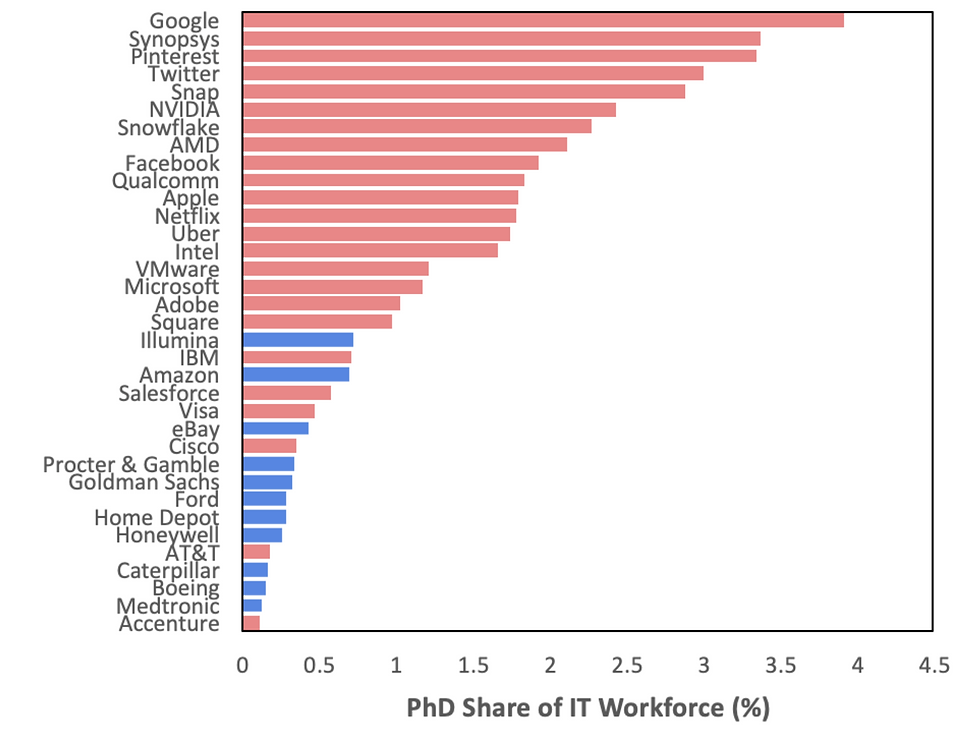
Source: S&P, Sparkline
We can backtest a simple investment strategy using this indicator. Each month, we buy the companies with the most educated IT workforce at that point in time. It turns out these companies have outperformed the market.
Since PhD IT workers tend to be concentrated in a few industries, we want to ensure that these results do not simply reflect the aggregate outperformance of these industries. Thus, we construct an industry-neutral strategy, which ensures the net exposure to each industry is zero. This portfolio looks quite different, with its long positions spread across industries. The industry-neutral strategy does well, implying that even within a given industry, the firms with the most skilled IT workforces have outperformed their peers.
Exhibit 13
Returns to Education

Source: S&P, Sparkline
Educational attainment provides a useful complement to other measures of innovativeness, such as R&D expenditure and patent grants. While this paper is focused only on IT human capital, this metric could be applied to many other disciplines (e.g., PhDs in biotech and other STEM fields).
Disruptive Talent
Information technology has been widely used in business for many decades. For example, the SQL database language first appeared in 1974 and by now has been almost universally adopted. The technologies that will ultimately prove disruptive are currently much earlier in their S-curves.
Therefore, in order to find companies investing in disruptive technologies, we must focus on a specific subset of IT skills. We could choose from a wide range of potential disruptive technologies. For example, we could use NLP to identify employees with specialized skills in cloud computing, computer vision, or robotics.
In this section, we will focus on artificial intelligence (AI) due to its potential to reshape almost every industry. AI enables machines to perform a wide range of advanced cognitive tasks, such as driving cars, speaking to customers, and processing massive datasets. If it fulfills its promise, it would disrupt workers everywhere.
We use companies’ hiring of workers skilled in AI to measure the extent of their investment in the technology. We identify AI roles based on either job titles (e.g., “machine learning engineer”) or required skills (e.g., “TensorFlow”). This can be done either using manual taxonomies or semi-supervised learning. Alekseeva et al (2020) and Babina et al (2020) study job postings and find (as expected) that the most requested AI skill is “machine learning” and the most common job requiring AI skills is “data scientist.”
Exhibit 14
Top AI Jobs and Skills

Source: Babina et al (2020), Alekseeva et al (2020), Sparkline
The share of jobs in AI has been increasing rapidly over the past decade, especially in the past few years. Most recently, demand appears to be outstripping supply, explaining the wage premium observed for employees with AI skills in otherwise similar roles.
Exhibit 15
AI Rising
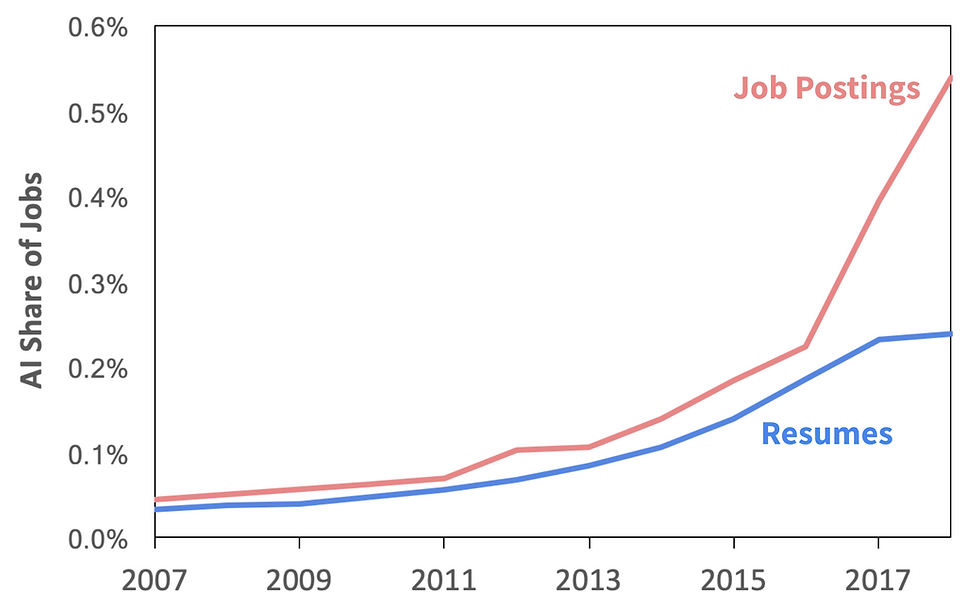
Source: Babina et al (2020), Sparkline
Compared to general software, AI is still in the early stages of adoption. As a result, AI talent is currently siloed in the IT and communications industries. However, we are gradually starting to see these skills diffuse across other industries. If AI fulfills its promise as a general purpose technology, we should expect this trend to continue.
Exhibit 16
AI Industry Concentration

Source: S&P, Sparkline
Within these sectors, the software and media subgroups have the greatest concentration of AI talent. However, there is massive dispersion. For example, Spotify has around 7% of their workforce in AI roles, which is over five times the industry average.
Exhibit 17
AI Employers in Software & Media
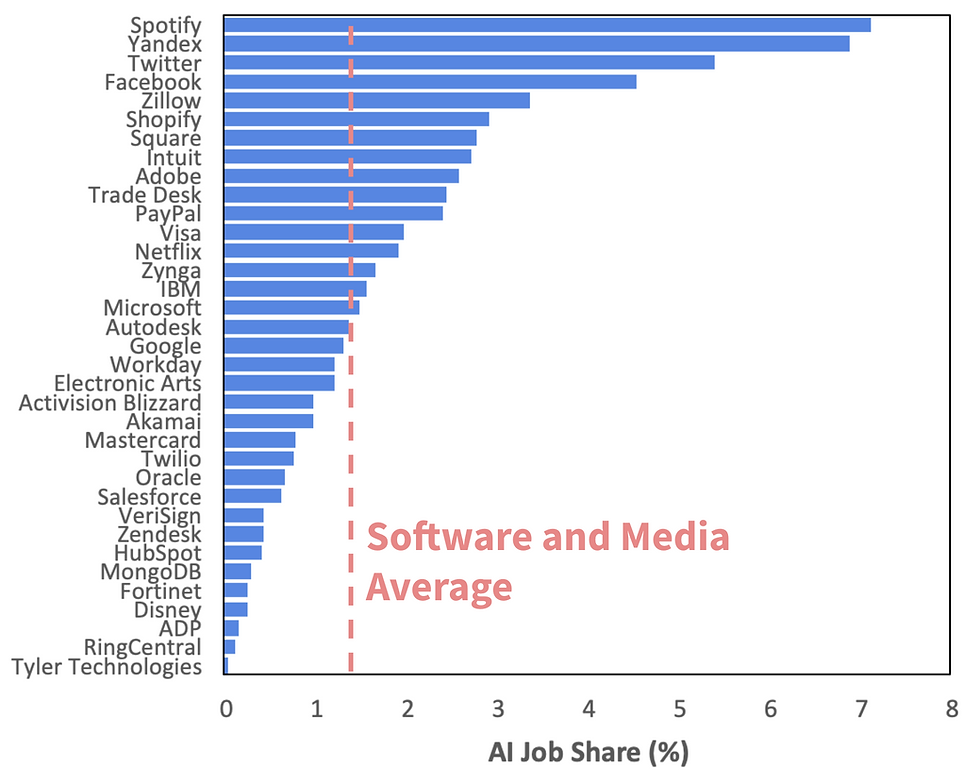
Source: S&P, Sparkline
Similar to our PhD example, we can backtest a strategy based on AI job share. We find that companies investing in AI talent have outperformed not only the market but also their industry peers.
Exhibit 18
AI Employers’ Outperformance
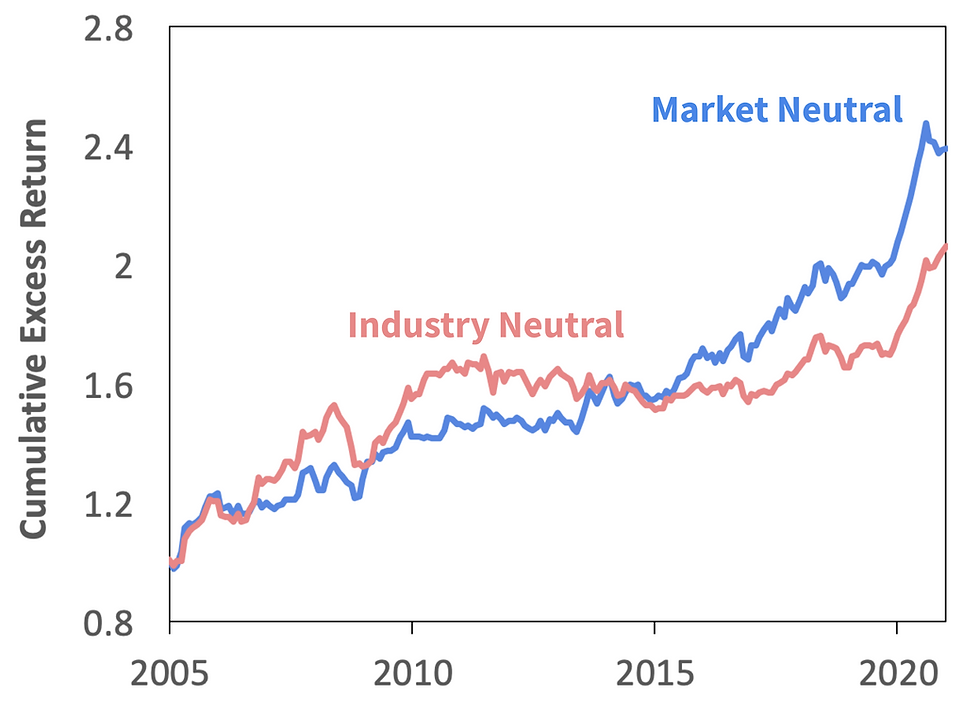
Source: S&P, Sparkline
While encouraging, the historical returns to AI human capital investment is not what we are playing for. AI is still in its infancy. The ultimate goal of investing in firms with strong AI talent is to benefit from the yet unrealized potential of future innovation.
Superstar Firms
The top AI researchers, such as Geoffrey Hinton and Yann LeCun, are employed by an elite cadre of tech giants and universities. Chuvpilo (2020) analyzed the affiliations of authors published in prestigious AI journals. Google holds the top spot, with over twice as many publications as second-ranked Stanford University.
Exhibit 19
AI Research Powerhouses
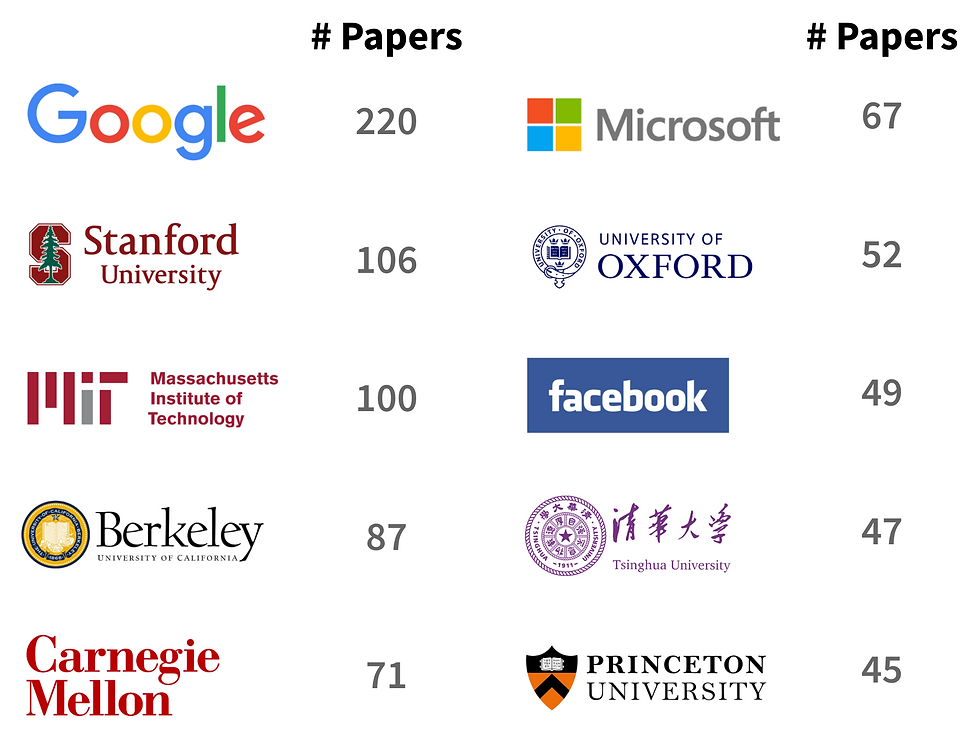
Source: Chuvpilo (2020), Sparkline
Of course, there is a big difference between research and implementation. Netflix did not invent video streaming nor Facebook the social network. The translation of scientific breakthroughs into commercial applications is a completely different skill. While most AI workers simply implement the creations of others, it turns out that even these frontline AI workers tend to be employed by the biggest firms in their respective industries.
Babina et al (2020) find that AI talent is concentrated not only in the largest but also the most profitable firms. They also find that firms that invest in AI have enjoyed stronger subsequent growth. This leads to positive feedback, whereby large, profitable firms can afford to invest in AI, allowing them to grow faster and further separate from the pack. This effect is not confined to the IT industry, but exists across industries. In addition, industries experiencing more aggregate AI talent investment have grown faster and experienced rising industry concentration.
Tambe et al (2020) conduct a similar analysis using the broader definition of IT workers. As with AI workers, they find the increase in IT employment has been concentrated in a subset of mega-cap companies. Over the past 30 years, the largest quartile of firms has driven almost all the increase in aggregate IT employment.
Exhibit 20
Superstar Firms
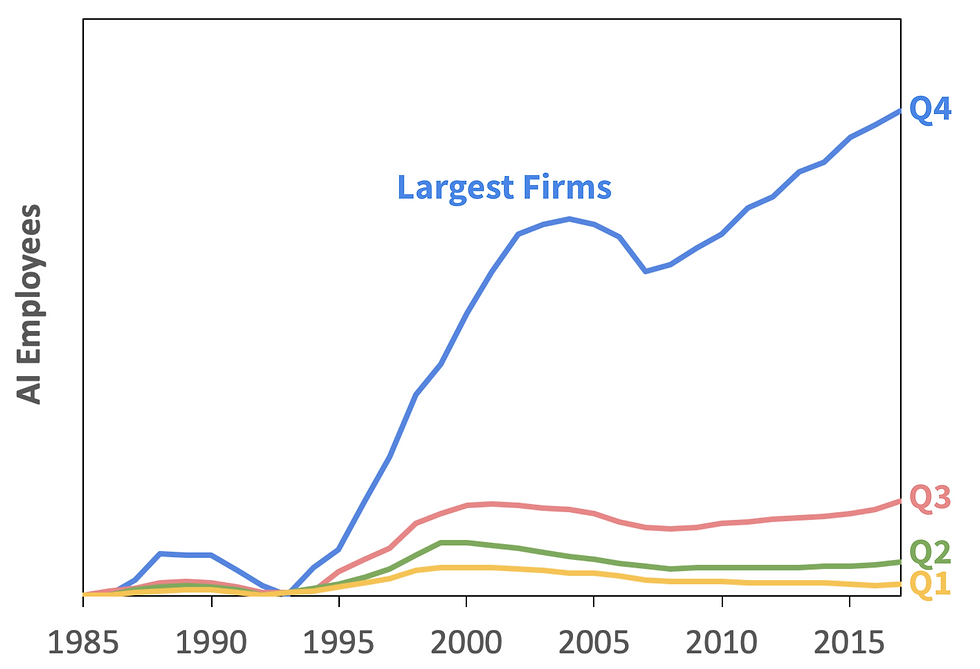
Source: Tambe et al (2020), Sparkline
In Monopolies Are Distorting the Stock Market (Sep 2020), we explained how the rise of superstar firms is framed by the dueling narratives of regulatory capture and technology. These new results lend support to the notion that the rise of superstar firms is primarily driven by the scale economies of technology. Big, profitable firms with lots of customer data can justify making large investments in technology, which allows them to further compound their advantage.
Firm Talent Profiles
Workforce Similarity
One interesting way to view a firm is as a bundle of its strategic resources (academics creatively call this the “resource-based view of the firm”). In the modern economy, human capital is the most important strategic resource. As the saying goes, “you’re only as good as your team!”
We can use labor market data to determine the human capital resources available to each employer. We do this by aggregating the skills possessed by individual employees to the level of the organization. This provides a picture of firms’ contrasting proficiencies. For example, Liu, Pant and Sheng (2020) show that, in addition to the obvious industrial differences, Amazon has a focus on customer service, Ford on Six Sigma and lean manufacturing, and IBM on solution architecture and integration.
Exhibit 21
Skill Profile Example
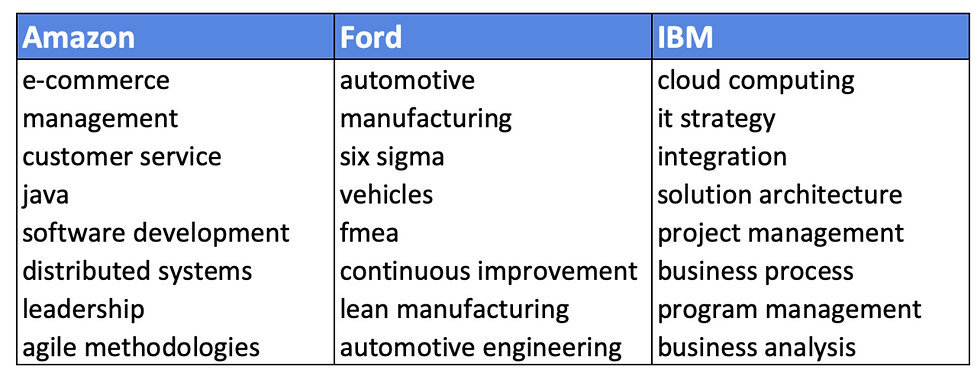
Source: Liu, Pant and Sheng (2020), Sparkline
In addition to skills, we can look at which types of roles companies tend to hire. We can then compare companies based on their intensity along each occupational dimension. For example, Amazon and Google are both invested heavily in engineering but have varying focuses on operations and media and communication.
Exhibit 22
Occupational Profile Example
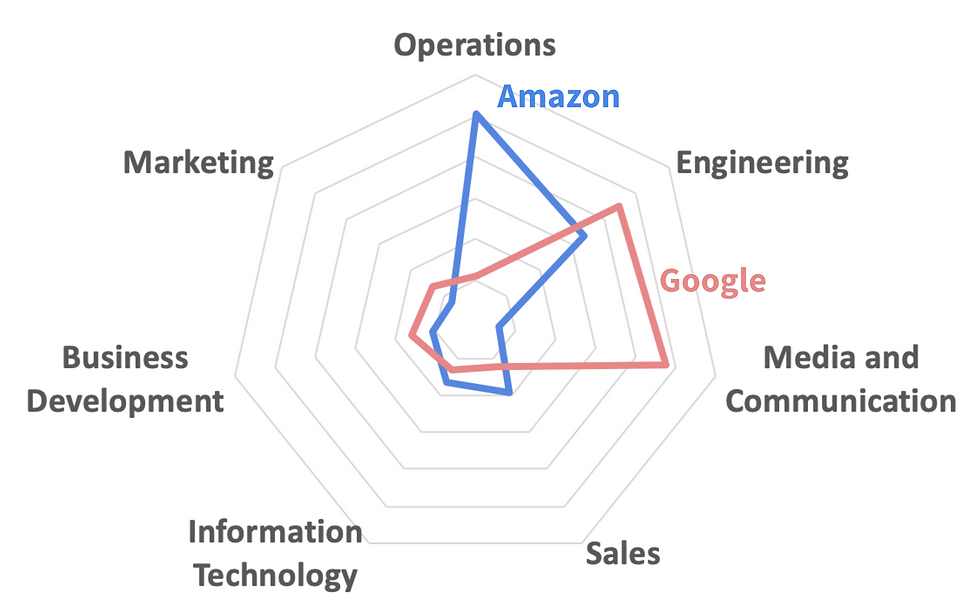
Source: LinkedIn, Sparkline
Educational qualifications provide a third dimension over which to compare companies. Some employers value the hard skills of STEM graduates while others prefer the generalist breadth of liberal arts majors. Some companies covet the deep domain specialization of PhDs, while others desire the managerial fluency of MBAs.
In Investment Management in the Machine Age (Jun 2019), we train “company embeddings” using 10-K business descriptions. These embeddings allow us to quantify the similarity of firms’ business models to each other. We can apply the same technique to firm talent profiles to compute similarity on the dimension of workforce composition.
The exhibit below provides a visualization of how companies cluster based on their workforce composition. We seed three groups with representative firms from autos (F, GM), tech (GOOG, CRM, ADBE), and banks (JPM, WFC). We also seed a fourth category with a single company: Tesla Motors.
Exhibit 23
Tesla Labor Market Map
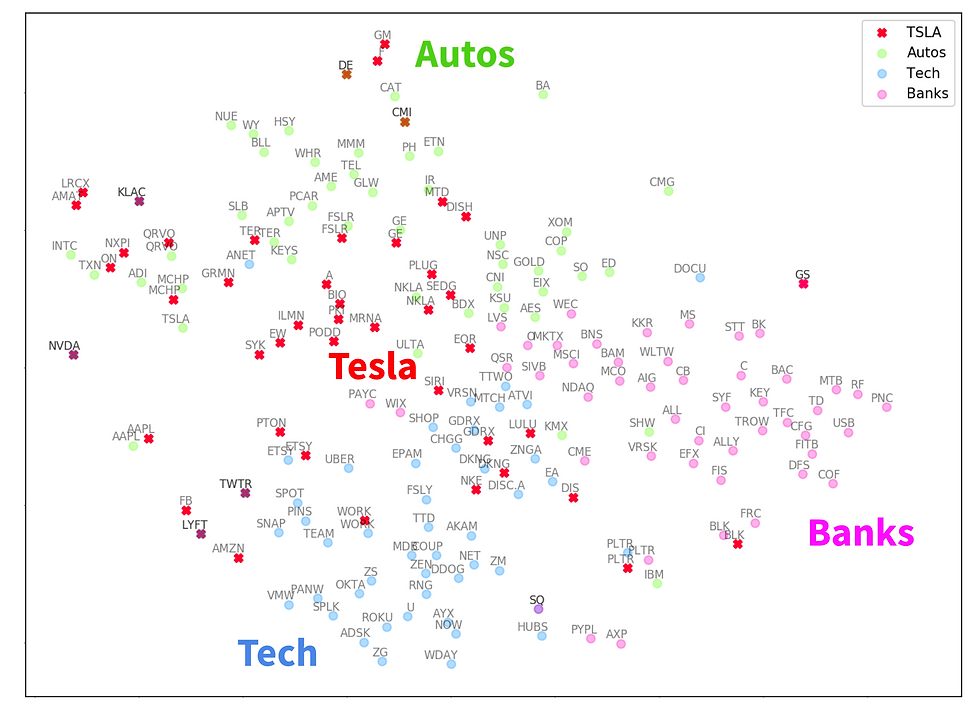
Source: Sparkline
As expected, companies tend to have similar workforces to other companies in their same industry. Auto companies need workers who can build cars and software companies need engineers who can code.
However, as we saw earlier with Amazon, Ford and IBM, not all skills are industry-specific. Tesla provides an interesting case study. Tesla employs not only thousands of people skilled in auto manufacturing but also thousands of software engineers. In this sense, its workforce is similar to those of both the auto and tech industries.
The Labor-Industrial Matrix
The most common way to define a company’s competitors is by the products they sell. This is the goal of industry classifications (e.g., GICS). Software companies sell software and auto companies sell cars. In economist jargon, industry competitors all sell into the same “product market.”
However, companies also compete in “factor markets” for the inputs to the production process. In the modern economy, the most important factor market is the labor market. Thus, labor profiles provide an alternate way to define competition between companies.
Liu, Pant and Sheng (2020) propose using a 2x2 matrix to analyze the overlap between companies on each of these two dimensions (i.e., labor input and product output). The next exhibit is a loose attempt to map Tesla’s competitors based on the level of overlap in labor and product markets.
Exhibit 24
Tesla’s Matrix
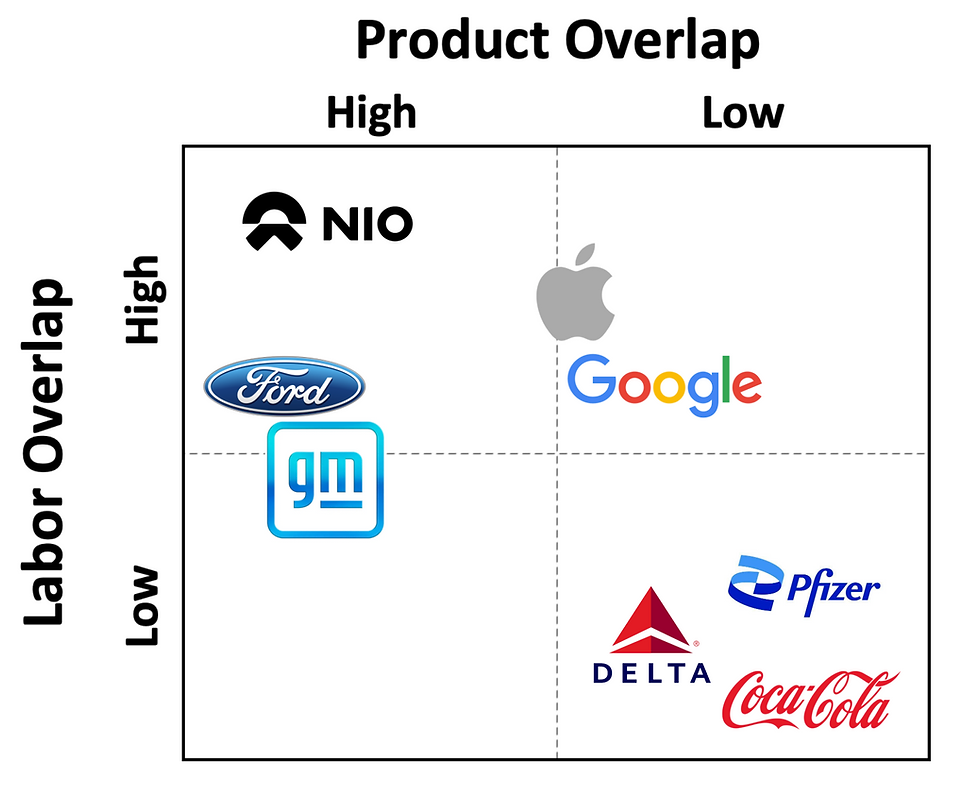
Source: Liu, Pant and Sheng (2020), Sparkline (for illustration only)
This provides a framework for competitive analysis. Given its first-mover advantage, Tesla doesn’t yet have a ton of direct competition (Nio in China and a few pre-production startups). However, competition is closing in on all fronts. Legacy automakers, such as Ford and GM, are investing heavily in electric vehicles. While further away, Tesla should also watch out for encroachment from established tech firms (e.g., Apple is rumored to be building an electric car).
Hidden Tech Companies
Nowadays, everyone wants to be a “tech company.” In 2017, Goldman Sachs’ CEO Lloyd Blankfein boldly declared “We are a technology firm.” WeWork, which operates co-working spaces, used the word “technology” more than 100 times in its prospectus, calling itself the “Google Analytics for space.”
But what does it actually mean to be a “tech company?” Ben Thompson of Stratechery writes: “Fifty years ago, what is a tech company was an easy question to answer: IBM was the tech company, and everybody else was IBM’s customers.” However, now that technology is crucial for all businesses, this binary definition is no longer very useful and the question is merely one of degree.
One way to determine the “centrality of software” to a company’s business is to examine its workforce. Companies that genuinely view technology as a key competitive advantage will commit significant resources to acquiring top engineering talent.
It turns out that Lloyd Blankfein’s declaration was more than just empty words. Goldman employs 10,000 developers, constituting 25% of its workforce. Technology is not only being used to streamline core operations but also underpins their online bank, Marcus. In contrast, in its 2019 prospectus, WeWork boasted that it employed “1,000 engineers, product designers and machine learning scientists.” But this comprised a mere 8% of its 12,500 person workforce.
Using our firm talent profiles, we can compute the degree to which each company’s workforce resembles that of a tech company. This provides a view of how central technology is to a company’s business model. We will focus exclusively on companies outside of the software and media industries, with the goal of identifying “hidden tech companies.” The following exhibit provides some representative results.
Exhibit 25
Tech Workforce Similarity

Source: Sparkline
Hidden tech companies rely heavily on technology in their production process but just so happen to sell products that for whatever reason are not classified as “technology.” For instance, Teladoc, Chegg, and Etsy are modern tech companies operating in healthcare, education, and retail, respectively. Also making appearances are legacy firms, such as Goldman Sachs, Best Buy and Nike, that have invested heavily in their digital transformations.
An analysis of the distribution of hidden tech companies by sector reveals that the financial sector contains the most tech-enabled companies. This includes both newer FinTech entrants and established players.
Exhibit 26
Hidden Tech by Sector
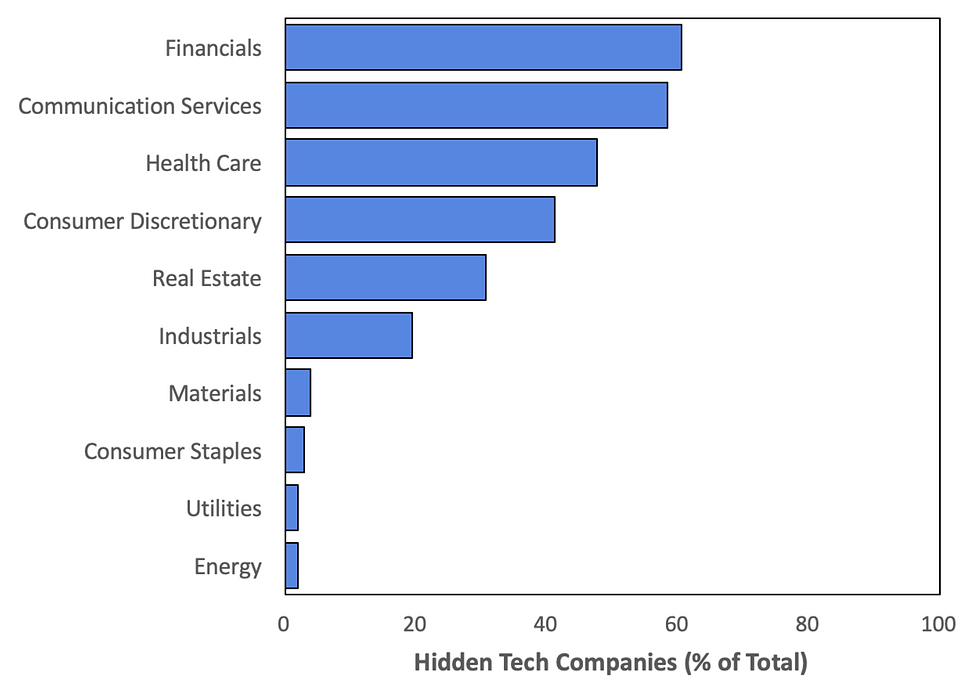
Source: Sparkline
Finally, we can create talent profile embeddings for each month going back in history. Each month, we form portfolios of hidden tech companies and calculate their subsequent performance compared to the market and industry peers.
Exhibit 27
Hidden Tech Outperformance
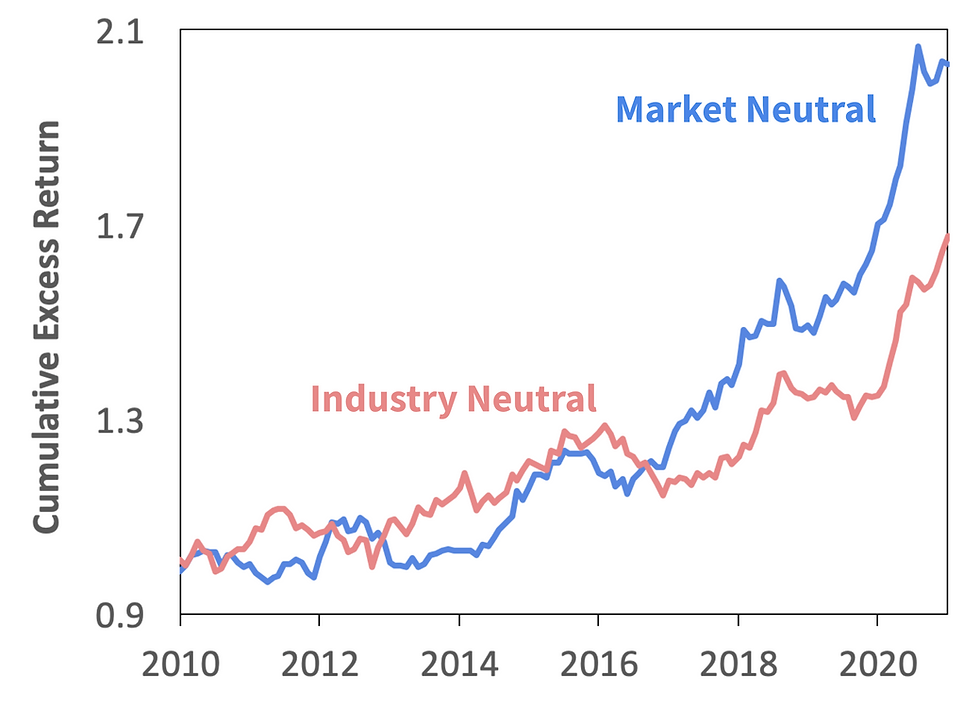
Source: Sparkline
Hidden tech companies have outperformed both the market and their industry competitors. These companies were early to embrace the disruptive potential of technology and their investments in building strong technical workforces have paid off handsomely.
The Future of Work
The End of Work
“We are being afflicted with a new disease of which some readers may not yet have heard the name, but of which they will hear a great deal in the years to come - namely, technological unemployment. This means unemployment due to our discovery of means of economising the use of labour outrunning the pace at which we can find new uses for labour.”
- John Maynard Keynes, Economic Possibilities for our Grandchildren (1930)
This prediction by the famous economist, John Maynard Keynes, frames centuries of popular anxiety over the death of work. The fear is that, as technological innovation continues its inexorable march, the tasks in which humans possess comparative advantage will converge toward zero.
Indeed, the machines are making great strides. In recent years, we have seen computers make inroads in many tasks requiring high-level cognitive and sensorimotor skills. Self-driving cars will eventually displace taxi and truck drivers. Meanwhile, robots threaten to displace workers in factories and fulfillment centers. A recent viral video reveals that robots can apparently now dance!
Exhibit 28
Dance Dance Revolution 🤖
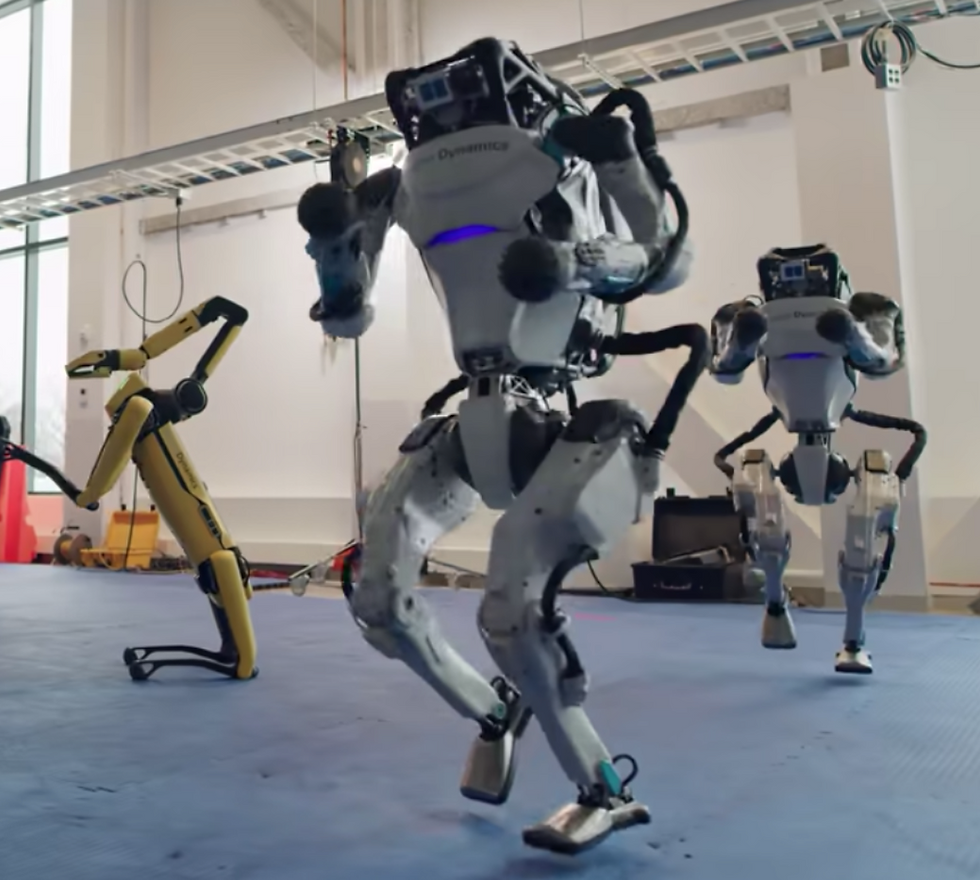
Source: Boston Dynamics
The economists Frey and Osborne (2013) conducted a more rigorous study to estimate the automation risk of 702 occupations based on their underlying skill requirements. The results are fairly intuitive: machines are more likely to displace telemarketers than choreographers.
Exhibit 29
Automation Risk
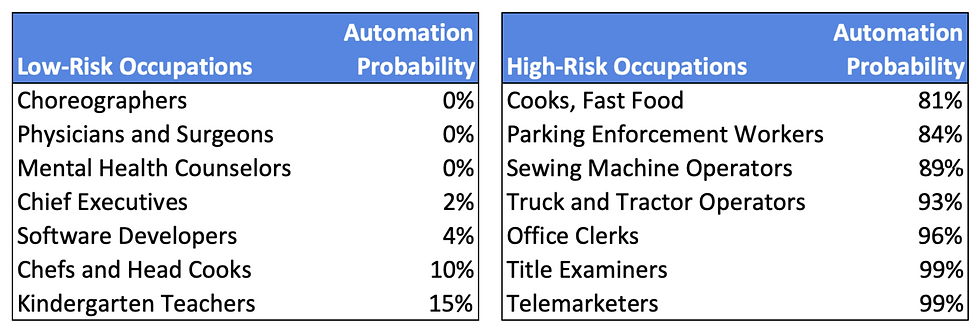
Source: Frey and Osborne (2013), Sparkline
In aggregate, they warn that 47% of U.S. employment is currently at risk of automation. Unfortunately, they also find that the safest jobs tend to require the greatest educational attainment. Full employment for a handful of highly paid PhDs and CEOs would provide little consolation in the face of rampant unemployment for everyone else.
The Luddite Fallacy
"[Creative Destruction is the] process of industrial mutation... that incessantly revolutionizes the economic structure from within, incessantly destroying the old one, incessantly creating a new one. This process of Creative Destruction is the essential fact about capitalism.”
- Joseph Schumpeter, Capitalism, Socialism and Democracy (1943)
On the other hand, technology has been disrupting the labor markets since the dawn of civilization. The invention of stone tools presumably displaced workers who were used to working with their hands. But it was only those workers that, for whatever reason, were unable to learn to use these new tools or find other work that ultimately faced structural technological unemployment.
The arc of human civilization suggests that we have nothing to worry about. In the past centuries, the economy has undergone many massive transformations. The share of U.S. employment in the agriculture sector fell from 40% in 1900 to 2% in 2010 due to productivity-enhancing technologies. However, for each job lost to the Schumperterian gale, another in a new industry rose to take its place. In the face of tumultuous change, the labor force participation rate has remained remarkably stable.
Exhibit 30
Death, Taxes, and 50% Employment?
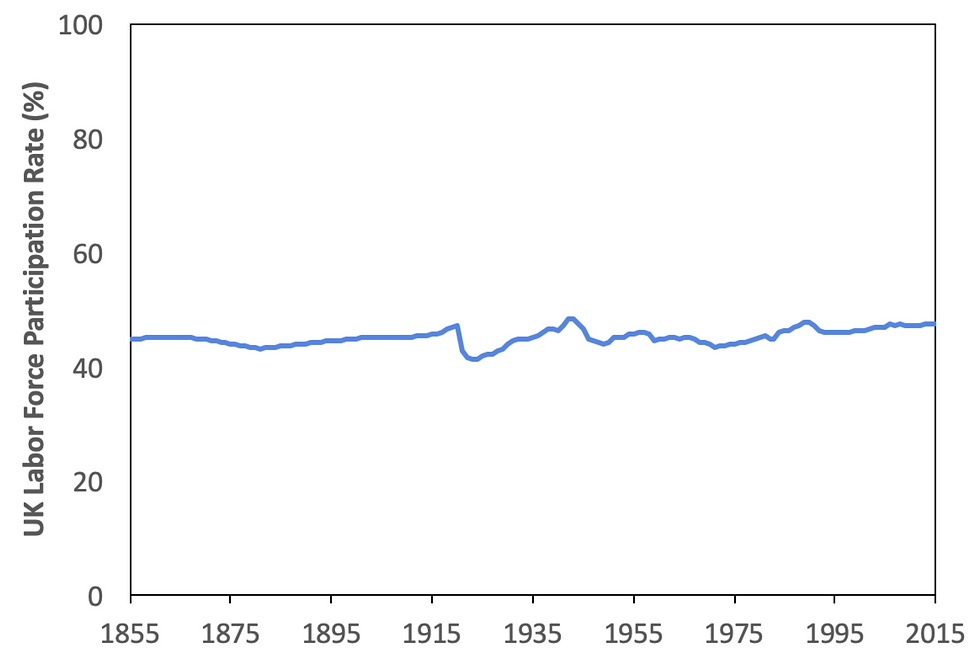
Source: Bank of England, Dorn (2015), Sparkline
We are now on the precipice of a third wave of automation, fueled by advances in artificial intelligence and robotics. There is no doubt that many jobs will be destroyed and new ones created. The question is only whether these futuristic advances will break the historical pattern of zero net job destruction. Will Keynes be right? Will this time be different?
Technological Inequality
“Technological progress is going to leave behind some people, perhaps even a lot of people, as it races ahead. [T]here’s never been a better time to be a worker with special skills or the right education, because these people can use technology to create and capture value. However, there’s never been a worse time to be a worker with only ‘ordinary’ skills and abilities to offer, because computers, robots, and other digital technologies are acquiring these skills and abilities at an extraordinary rate.”
- McAfee and Brynjolfsson, The Second Machine Age (2014)
While Keynes was primarily concerned with the net impact of technology on employment, we believe a more urgent problem is the rise in technology-led inequality.
This can be seen if we split the labor force on the dimension of “routineness.” Routine jobs involve well defined repetitive tasks, which are ripe for automation. These jobs can be both manual (e.g., production work) and cognitive (e.g., clerical work).
Exhibit 31
Job Classification
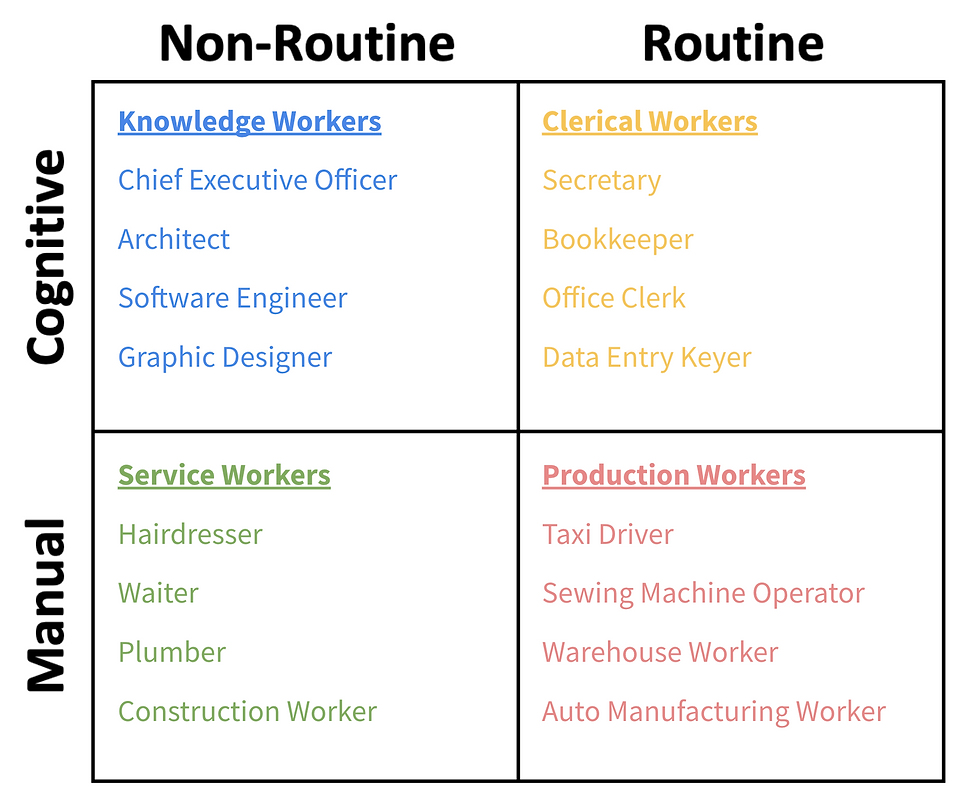
Source: Sparkline
Since the 1980s, nearly all net job growth has occurred in non-routine jobs. Creative destruction has continued to operate, but with an increasingly uneven hand.
Exhibit 32
The Death of the Routine

Source: US Bureau of Labor Statistics, Sparkline
The information age has been doubly unkind toward workers in routine jobs. First, machines directly compete with them for jobs in both the factory and office. Second, modern communication technologies have made it easier for companies to offshore routine manufacturing and IT roles, leading to further competition from lower-paid workers in developing countries.
The problem is that the two growing sectors are at opposite ends of the income distribution. Non-routine manual jobs tend to be in the service industry, requiring less training and education and offering lower average pay. On the other hand, non-routine cognitive jobs are “knowledge work” with high skill requirements and compensation.
Exhibit 33
Job Polarization
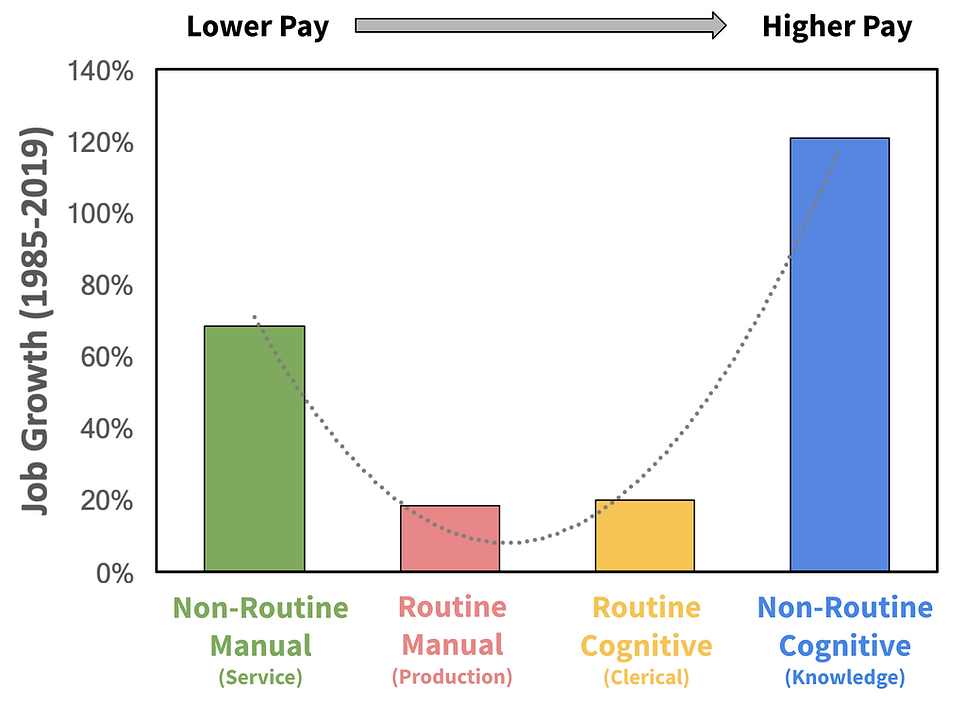
Source: US Bureau of Labor Statistics, Sparkline
While there are many reasons for the death of the American middle class, technology is one of the most important. Automation is eliminating jobs in the middle of the skill and income distribution, hollowing out the middle class.
This is especially concerning in light of the ongoing Covid-19 pandemic. Ding and Molina (2020) show that the pandemic has already disproportionately affected workers in jobs at risk of automation, such as toll collectors, call center workers, hotel receptionists, and meatpacking workers.
Much of this job disruption may turn out to be permanent. Jaimovich and Siu (2012) find lasting devastation in routine jobs in the wake of the past few recessions. Furthermore, Modestino et al (2016) and Hershbein and Kahn (2016) find that companies took advantage of the 2008 recession to upskill their workforces, restructuring production in a way that favors higher-skilled workers. We may very well be in for a repeat of these recent experiences.
Race Against the Machine
One interesting framing of the debate is as a race between technology and education (Goldin and Katz (2007)). The idea is that technological progress constantly increases the skill requirements for labor. However, since 1980, the supply of educated workers has failed to keep pace with innovation. This supply-demand imbalance has led to a rising premium for college-educated workers, creating economic inequality.
Exhibit 34
The College Premium
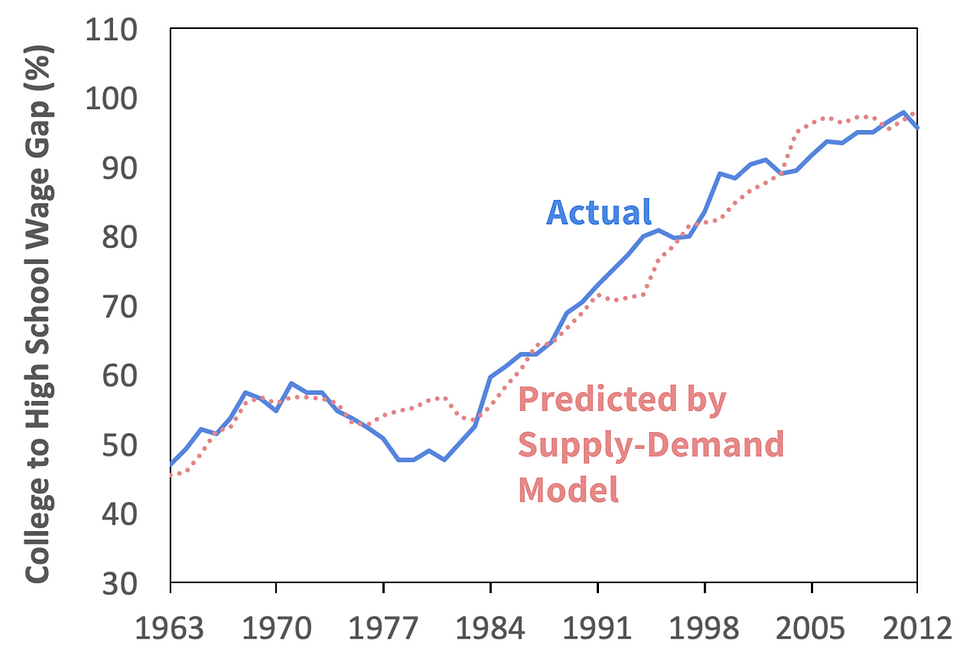
Source: Autor (2014), Sparkline
We believe this supply-demand narrative is broadly correct with one clarification. The so-called “skill gap” is less about a shortage of college graduates than a shortage of workers that can serve as technological complements. College degrees are but one proxy for the skills demanded in the rapidly changing modern economy.
Our overarching belief is that humans should seek to complement machines rather than compete with them. We should specialize in areas in which we have comparative advantage over machines. This includes interpersonal communication, creativity, problem solving, empathy, and cross-domain integration.
If we view jobs as bundles of tasks, the logical endgame is for jobs to be rebundled into task combinations based on human comparative advantage. For instance, in the decades after the introduction of the ATM, the number of bank tellers actually grew. While ATMs did largely automate the task of dispensing cash, with this routine task out of the way, bank tellers were able to focus on the more human part of their jobs as “relationship bankers.”
The Future of Human Capital
Our future depends on the investments we make in human capital. As technology races ahead, we must educate and train our labor force to be able to add value alongside increasingly advanced machines.
First, broadening access to higher education is a critical remedy for the shortfall in skilled workers. However, as our college system suffers from spiraling costs and unnecessary bundling and credentialism, alternative pathways such as a robust vocational training system are likely to be a critical part of the solution.
Second, our educational system should focus on imparting skills in which humans have comparative advantage. We should create a workforce that is able to work in partnership with the machine. Let’s teach creativity, empathy, and teamwork, not rote memorization.
Finally, we need to foster an orientation toward lifelong learning. The process of creative destruction is accelerating. Unfortunately, the human capital investment cycle is extremely front-loaded. People devote their first 16 to 24 years of life to learning and the rest to doing. However, due to the shrinking half-life of knowledge, workers cannot rely exclusively on information learned in formal education. The private sector must provide opportunities for employees to continually improve their skills.
Conclusion
Most investors evaluate companies based on their tangible attributes, such as book value and physical assets. However, these metrics are increasingly irrelevant today. Investors need to reorient their focus toward intangible assets, which are the drivers of modern value creation.
Human capital is among the most important intangible assets. The investments that companies make in their workforces say a lot about them. We can learn how well positioned they are for the digital age, the extent of their investment in specific disruptive technologies, and the centrality of technology to their business model.
As the growth of human knowledge accelerates, the fortunes of companies will increasingly depend on making continual investments in highly skilled and adaptable workforces. Labor market data provides investors with an invaluable tool to determine which companies are making these necessary investments.
Disclaimer
This paper is solely for informational purposes and is not an offer or solicitation for the purchase or sale of any security, nor is it to be construed as legal or tax advice. References to securities and strategies are for illustrative purposes only and do not constitute buy or sell recommendations. The information in this report should not be used as the basis for any investment decisions.
We make no representation or warranty as to the accuracy or completeness of the information contained in this report, including third-party data sources. The views expressed are as of the publication date and subject to change at any time.
Hypothetical performance has many significant limitations and no representation is being made that such performance is achievable in the future. Past performance is no guarantee of future performance.